17 Enhancing Operational Data Collection and Risk Management at Hydroelectric Dams by Using Digital Twins:
Harikrishna Chalamalasetty
Introduction:
Renewable energy sources are essential to meet today’s substantial energy needs. The primary renewable options include wind, hydropower, nuclear, and biomass. Among these, hydropower stands out as the most adaptable and reliable source, being relatively more affordable and accessible. In addition to generating hydroelectric power, hydropower dams are also beneficial for irrigation purposes [1]. However, their large-scale operation involves significant challenges and risks, necessitating efficient and safe management practices. The evolution of digital technologies presents new opportunities for enhancing the operational efficiency and risk management of these critical structures. Among these technologies, digital twin technology stands out as a promising solution.
Digital twins play a crucial role in optimizing the operation, maintenance, and overall performance of hydropower facilities. They enable performance monitoring and analysis by allowing operators to analyze real-time data to track a facility’s performance and detect deviations from optimal operating conditions. Through predictive maintenance, digital twins can forecast equipment failures and maintenance needs by examining historical and real-time sensor data, facilitating timely maintenance activities, reducing downtime, and preventing costly unplanned outages. Furthermore, digital twins help optimize energy production by analyzing data and simulating various operating conditions, enabling operators to adjust turbine settings, manage water reservoir levels, and align energy generation with demand fluctuations. Additionally, they enhance resource management and environmental impact assessment by modeling water inflows, reservoir levels, and environmental factors, which helps in minimizing water usage and evaluating the effects of different operational strategies on the environment. This allows operators to make informed decisions that reduce negative impacts [2].
Rationale for Studying the Case:
Hydroelectric dams are essential components of our energy infrastructure, providing clean and renewable energy to communities worldwide. However, their efficient and safe operation is paramount, given the potential risks associated with such large-scale structures. By studying the methods and technologies used in operational data collection, particularly through the lens of digital twin technology, we can gain valuable insights into how to optimize the performance and safety of hydroelectric dams. This investigation is crucial not only for ensuring the reliable supply of electricity but also for minimizing environmental impacts and protecting surrounding ecosystems. Ultimately, the rationale for studying this case lies in the need to enhance our understanding of data collection processes to bolster the resilience and sustainability of hydroelectric dams in the face of evolving challenges.
Motivation for the Problem/Research Question:
The motivation for delving into this problem stems from the critical role that hydroelectric dams play in our energy infrastructure and the inherent risks associated with their operation. Efficient data collection and robust risk management practices are essential for mitigating these risks and ensuring the continued operation of dams. By focusing on digital twin technology, which offers a promising avenue for improving data collection and risk management, we aim to address current gaps and identify opportunities for enhancement. This research question is motivated by the need to optimize dam operations, maximize energy output, and minimize potential disruptions, ultimately contributing to the sustainable management of our energy resources.
Purpose:
The purpose of this case study is multifaceted. Firstly, we aim to examine existing practices and technologies used in operational data collection at hydroelectric dams, with a specific emphasis on digital twin technology. By doing so, we seek to identify areas for improvement and innovation, ultimately enhancing the efficiency and effectiveness of data collection processes. Secondly, we aim to explore how digital twin technology can be seamlessly integrated into the operational stage of hydroelectric dams to facilitate proactive risk management. Through this investigation, we aspire to provide actionable recommendations for optimizing data-driven operational management and risk mitigation strategies.
Focus:
Our study will focus on evaluating the technologies and systems employed for operational data collection and risk management at hydroelectric dams, with a keen emphasis on digital twin technology. This includes an in-depth analysis of the sensors, monitoring equipment, remote sensing tools, and data management platforms utilized to track critical parameters such as water levels, flow rates, structural integrity, and environmental impacts. Additionally, we will explore the integration of digital twin technologies into these processes, assessing their effectiveness, accuracy, and potential for enhancing risk management practices. Through this focused approach, we aim to uncover insights that will inform strategies for optimizing data collection efficiency and integrating risk management seamlessly into dam operations.
Unit of Analysis:
The unit of analysis is to be hydroelectric dams and the use of digital twin technology to enhance operational data collection and risk management at these facilities.
Detailed Description of the Facts Related to the Case:
Hydroelectric dams rely on a myriad of sensors, meters, SCADA systems, and monitoring equipment to collect vast amounts of data related to electricity generation, water levels, turbine performance, and environmental conditions. Digital twin technology offers a virtual representation of these physical assets, enabling real-time simulation, analysis, and predictive modeling. By integrating data from various sources into a digital twin framework, operators can gain valuable insights into dam operations, identify potential risks, and make informed decisions to mitigate them. This detailed description provides the foundation for understanding the complexities involved in data collection and risk management at hydroelectric dams.
Description of the Data Collected:
Our data collection efforts will encompass a comprehensive range of information related to the types of sensors, monitoring technologies, and data management platforms used at hydroelectric dams. We will gather quantitative data on the frequency, accuracy, and reliability of data collection processes from the literature reviews. Additionally, we will focus on the integration of digital twin technology into the operational data collection framework, exploring its capabilities and potential for enhancing risk management practices. This detailed description of the data collected ensures a holistic understanding of the factors influencing data collection and risk management at hydroelectric dams.
In addition to the literature review, we will conduct a detailed case study analysis focusing on select hydroelectric dams. Through case studies, we will gather empirical data on the implementation of sensors, monitoring technologies, and data management platforms, as well as the integration of digital twin technology where applicable. By examining real-world scenarios, we aim to gain insights into the practical challenges, successes, and lessons learned in data collection and risk management within specific hydroelectric dam settings.
By employing both qualitative and quantitative sources, our research aims to validate trends, enhance understanding, and provide comprehensive insights into the complexities surrounding data collection and risk management in hydroelectric dams. Ultimately, this integrated approach will inform future practices and decision-making in this critical domain, contributing to improved operational efficiency and safety in hydroelectric dam management.
Literature Review:
The digital twin concept aimed to create a virtual representation mirroring the current and future state of physical assets throughout their operational lifecycle. At its core, a comprehensive digital twin requires the physical asset itself, virtual models that can simulate the asset’s behavior and condition, and data connections to automatically update the virtual model with information from the operational asset. However, defining architectures to realize reliable digital twins for complex systems like hydroelectric generating units remains an active area of research. Proposed approaches consolidate diverse data sources – design specifications, real-time sensor data, operational histories, and maintenance records – into integrated data lake environments. Physics-based models capturing governing equations, data-driven models leveraging machine learning on operational data, and hybrid techniques combining both paradigms are developed for key components and integrated into a multi-model framework.
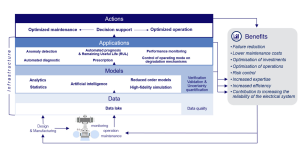
Key application modules span anomaly detection by monitoring deviations from predicted behavior, diagnostic analyses to link anomalies to root causes and failure modes, prognostic models for remaining useful life predictions, and prescriptive analytics to recommend optimal maintenance and operational strategies. However, realizing reliable digital twins faced challenges like accurately modeling complex multi-physics phenomena, addressing data quality and scarcity issues through techniques like synthetic data generation, and ensuring interpretability of AI/ML model insights. Looking ahead, the goal was to transition from asset-level digital twins to integrated ecosystems representing interconnected industrial systems.
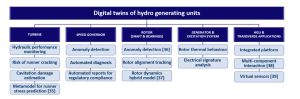
Despite the challenges, digital twins hold immense potential to optimize asset operations and maintenance practices across industries. As research continues to overcome bottlenecks like modeling complexities and data quality issues, fostering cultural adoption of this technology as a trusted decision support tool remains a crucial goal. With early proof-of-concept implementations demonstrating promising results, the path ahead aims to democratize digital twins, enabling their seamless integration into organizational workflows to efficiently leverage their insights and drive effective decision-making for improved asset utilization and reliability. [3]
Case Study:
Tooliqa, an industrial AI company, developed a digital twin for a major hydropower plant owned by a leading European energy company. The goal was to improve operational efficiency, enable predictive maintenance, and optimize power generation.
A digital twin is a virtual replica of a physical asset or system that uses real-time data to simulate and optimize its performance. In the case of a hydro power plant, the digital twin integrates data from various sources like sensors, maintenance records, weather forecasts, and hydrological models to create a comprehensive virtual model. A major challenge in the industry was faced by a hydropower generation company seeking a solution for continuous, real-time remote monitoring and full accessibility of their entire plant.
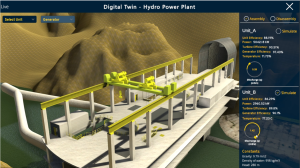
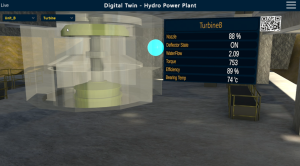
Key Components
The digital twin consists of several interconnected modules:
Asset Model: A 3D virtual representation of the physical hydro power plant components like dams, turbines, generators, and transmission lines. This model captures the asset’s geometry, materials, and behavior.
Sensor Data Integration: Real-time data from sensors monitoring water levels, flow rates, vibrations, temperatures etc. is continuously fed into the digital twin to reflect the actual operating conditions.
Physics-based Simulations: Advanced simulations based on fluid dynamics, structural mechanics, and electromechanical principles model the complex interactions within the hydro plant systems.
Analytics and Optimization: Machine learning algorithms analyze the integrated data to identify patterns, predict performance, and optimize operations by adjusting parameters like water flow, turbine angles, and generator output.
Key Benefits
The digital twin offers several benefits for hydropower plants:
Predictive Maintenance: By monitoring asset health and simulating various scenarios, maintenance can be scheduled proactively before failures occur, reducing downtime.
Operational Efficiency: Optimizing water usage, turbine settings, and generator output based on real-time conditions maximizes efficiency and power generation.
Risk Mitigation: Simulating extreme events like floods or earthquakes helps identify vulnerabilities and develop mitigation strategies.
Lifecycle Management: The digital twin provides a comprehensive view of the plant’s lifecycle, enabling better planning for upgrades, retrofits, or decommissioning.
In conclusion, the implementation of a digital twin for hydropower plants represents a significant advancement in asset management and operational optimization. By leveraging real-time data integration, sophisticated simulations, and analytics, the digital twin offers a holistic view of the plant’s performance and health. This technology enables predictive maintenance strategies, enhances operational efficiency, mitigates risks associated with extreme events, and facilitates informed decision-making throughout the plant’s lifecycle. Ultimately, the digital twin not only enhances the reliability and performance of hydropower plants but also contributes to sustainability and resilience in the energy sector. [4]
Discussion of the Patterns/Theories Found:
The discussion of patterns/theories involves analyzing recurring trends or frameworks observed during the study are:
Data Integration and Consolidation:
This pattern highlights the importance of consolidating diverse data sources, such as design specifications, real-time sensor data, operational histories, and maintenance records, into a unified data environment. This integration of data from multiple sources enables a comprehensive and holistic representation of the physical asset, facilitating better decision-making and risk management.
Multi-Model Approach:
Digital twins leverage a combination of physics-based models, data-driven models (e.g., machine learning), and hybrid techniques to capture the complex behavior and dynamics of hydroelectric power generation units. This multi-model approach allows for accurate simulations, predictive analytics, and a deeper understanding of the underlying systems.
Lifecycle Perspective:
Digital twins aim to mirror the current and future state of physical assets throughout their entire operational lifecycle, from design to decommissioning. This lifecycle perspective enables proactive risk management, predictive maintenance, and optimized operational strategies, ensuring the long-term sustainability and reliability of hydroelectric dams.
Interconnected Systems:
The literature review suggests a transition from asset-level digital twins to integrated ecosystems representing interconnected industrial systems. This pattern aligns with the larger scheme of the Industrial Internet of Things (IIoT) and Industry 4.0, where digital twins play a crucial role in optimizing and managing complex interconnected systems.
Connection to the Larger Scheme of Things:
The adoption of digital twin technology for hydroelectric dams represents a convergence of several broader trends, including industrial digital transformation, sustainable energy practices, critical infrastructure resilience, and interdisciplinary collaboration. Digital twins align with the principles of Industry 4.0, leveraging advanced technologies like the Internet of Things (IoT), artificial intelligence (AI), and virtual modeling to optimize operations and improve efficiency. Their implementation contributes to environmental sustainability by enabling proactive risk management, predictive maintenance, and optimized operations, minimizing the ecological impact of hydropower generation. Additionally, digital twins support the reliable functioning of hydroelectric dams, which are classified as critical infrastructure, enhancing energy security and economic development. As the global energy landscape shifts towards renewable sources, digital twins can facilitate the integration of hydroelectric power with other clean energy technologies, aiding the energy transition.
Conclusion:
The integration of digital twin technology into hydroelectric dam operations represents a significant leap forward in the energy sector, enhancing the efficiency, reliability, and sustainability of these critical infrastructures. Digital twins provide a virtual replica of physical assets, enabling real-time monitoring, simulation, and predictive maintenance. By consolidating data from various sources, such as real-time sensor data, operational histories, and maintenance records, digital twins offer operators valuable insights into the performance and health of hydroelectric facilities. This comprehensive data integration facilitates proactive risk management, optimizing operational strategies, and improving overall efficiency in power generation. The case study of Tooliqa’s digital twin implementation at a major hydropower plant exemplifies these benefits, showcasing improvements in operational efficiency, predictive maintenance, and risk mitigation.
Furthermore, the application of digital twins aligns with broader trends in industrial digital transformation and sustainable energy practices. As part of the Industry 4.0 paradigm, digital twins leverage advanced technologies like IoT, AI, and virtual modeling to optimize complex infrastructures. Predictive maintenance enabled by continuous monitoring and advanced simulations helps preempt equipment failures, reducing downtime and costs. Optimizing operational parameters enhances energy production efficiency, while modeling environmental factors supports sustainable resource management and environmental impact assessment. Ultimately, the adoption of digital twin technology will be crucial in ensuring the optimal performance and longevity of hydroelectric facilities, contributing to a resilient and sustainable energy future.
References:
[1].https://www.researchgate.net/publication/330843401_Hydropower_Dams_Environment_and_Politics
[2].https://www.pnnl.gov/projects/digital-twins-hydropower
[3]. https://www.researchgate.net/publication/375890702_Application_of_the_Digital_Twin_Concept_for_Operation_and_Maintenance_of_Hydro_Generating_Units
[4]. https://www.tooli.qa/insights/hydro-case-study
Media Attributions
- Fig: Outline of digital twin for asset operation and maintenance
- Fig: Components and Applications involved in digital twins for hydroelectric power generation units.
- Fig: Simulated view of the entire hydropower plant with real-time IoT data
- Fig: Simulated view of the individual units